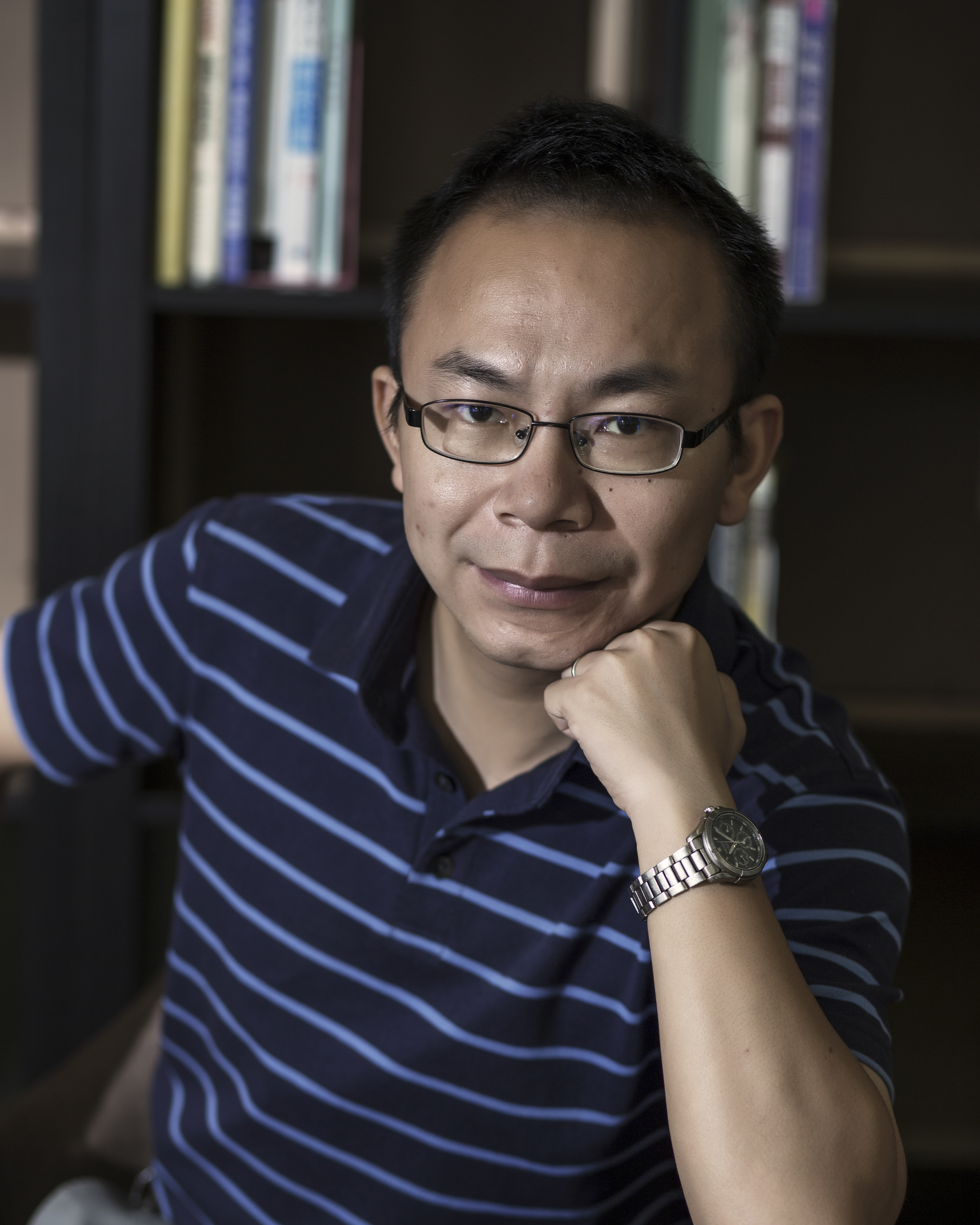
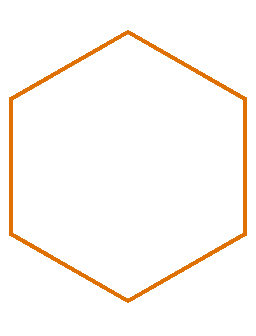
邹磊 (北京大学 教授)
Lei Zou received his BS degree and Ph.D. degree in Computer Science at Huazhong University of Science and Technology (HUST) in 2003 and 2009, respectively. He received a CCF (China Computer Federation) Doctoral Dissertation Nomination Award in 2009, won Second Class Prize of CCF Natural Science Award in 2014 and Second Class Prize of Natural Science of the Ministry of Education, China in 2017. Since September 2009, he joined Institute of Computer Science and Technology (ICST) of Peking University (PKU) as a faculty member. He has been a professor in PKU since August 2017. Before joining PKU, he visited Hong Kong University of Science and Technology in 2007 and University of Waterloo in 2008 as a visiting scholar. His recent research interests include graph databases, knowledge graph, particularly in graph-based RDF data management. He has published more than 50 papers, including more than 30 papers published in reputed journals and major international conferences, such as SIGMOD, VLDB, ICDE, TODS, TKDE, VLDB Journal. Lei Zou’s research is supported by NSFC-Young Excellent Talent Project and National Key Research and Development Program of China.
报告题目: Natural Language Question Answering over Knowledge Graph
报告时间: 09:10—09:55
报告摘要:
AS more and more structured data become available on the web, the question of how end users can access this body of knowledge becomes of crucial importance. As a de facto standard of a knowledge base, RDF (Resource Description Framework) repository is a collection of triples, denoted as
In this talk, I first review two categories of existing methods on natural language question answering (Q/A) over RDF knowledge graph---one is IR (Information Retrieval)-based and the other one is called semantic parsing method. Then, I will talk about our RDF Q/A system (gAnswer), which is based on graph matching-based technique. The most challenge to RDF Q/A task is the ambiguity of natural language question sentence. The contribution of our method is that we combine the disambiguation and query evaluation in a uniform process, i.e., we push down the disambiguation into the query evaluation stage. Based on the query results over RDF graphs, we can address the ambiguity issue efficiently. gAnswer joined QALD-6 knowledge graph Q/A competition (hosted by ESWC) and it won the second place in the Q/A precision. We host an online demo of our system at ganswer.gstore-pku.com and the relevant source codes are released at Github.
朱其立 (上海交通大学 教授)
Kenny Qili Zhu is a tenured Professor of computer science at Shanghai Jiao Tong University. He graduated with B.Eng (Hons) in Electrical Engineering in 1999 and PhD in Computer Science in 2005 from National University of Singapore. He was a postdoctoral researcher and lecturer from 2007 to 2009 at Princeton University. Prior to that, he was a software design engineer at Microsoft, Redmond, WA. From Feb 2010 to Aug 2010, he was a visiting professor at Microsoft Research Asia in Beijing. Kenny's current research interests are natural language processing and knowledge engineering. He has published extensively in databases, AI and programming languages at top venues such as ACL, EMNLP, AAAI, IJCAI, SIGMOD, KDD, CIKM, ICDE. He has served on the PC of AAAI, IJCAI, WWW, CIKM, ECML, COLING, SAC, WAIM, APLAS and NDBC, etc. His research has been supported by NSF China, MOE China, Microsoft, Google, Oracle, Morgan Stanley and AstraZeneca. Kenny is the winner of the 2013 Google Faculty Research Award and 2014 DASFAA Best Paper Award.
报告题目:Matching Questions and Answers in Dialogues from Online Forums
报告时间:10:05—10:50
报告摘要:
Matching question-answer relations between two turns in conversations is not only the first step in analyzing dialogue structures, but also valuable for training dialogue systems. This talk presents a QA matching model considering both distance information and dialogue history by two simultaneous attention mechanisms called mutual attention. Given scores computed by the trained model between each non-question turn with its candidate questions, a greedy matching strategy is used for final predictions. Because existing dialogue dataset such as the Ubuntu dataset are not suitable for the QA matching task, we further create a dataset with 1,000 labeled dialogues and demonstrated that our proposed model outperforms the state-of-the-art and other strong baselines, particularly for matching long-distance QA pairs.
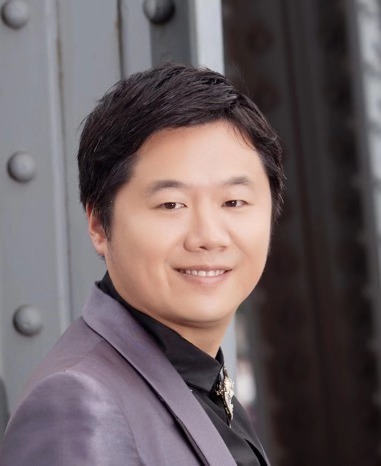
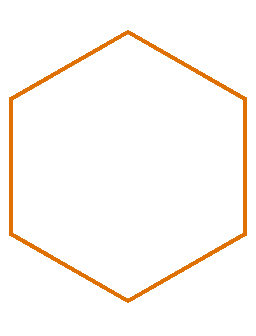
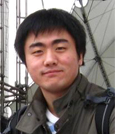
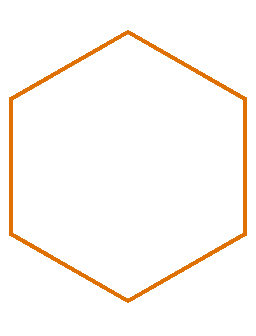
程龚 (南京大学 副教授)
程龚,南京大学计算机科学与技术系副教授、江苏省“六大人才高峰”高层次人才。目前主要面向智能软件系统,研究语义网与知识图谱技术,研究主题包括语义搜索、数据摘要、智能问答等。主持国家重点研发计划课题、国家自然科学基金面上项目等多个项目课题。在WWW、AAAI、IJCAI、TKDE等会议期刊上发表论文70余篇,获ISWC最佳论文提名2次、COLING最佳论文提名1次,论文总引用2000余次。现任中国计算机学会系统软件专委委员、中国中文信息学会语言与知识计算专委委员、江苏省人工智能学会知识工程与智能服务专委副秘书长,担任过ISWC短文程序委员会主席、CCKS领域主席等职务。
报告题目:知识图谱中的关联搜索
报告时间:10:50—11:25
报告摘要:
TBA
钱铁云 (武汉大学 教授)
Tieyun Qian is a full professor in School of Computer Science, Wuhan University, China. She received her PhD degree in Computer Science from Huazhong University of Science and Technology, China. She has been researching into text mining, Web mining, and natural language processing, with over 60 publications on leading conferences and journals like ACL, CIKM, SIGIR, TOIS, and TKDD. She is a member of CCF, ACM, IEEE, and ACL. She has served as the program committee member of many premium conferences including WWW, IJCAI, AAAI, CIKM, and DASFAA.
报告题目:Understanding User Generated Information
报告时间:13:30—14:15
报告摘要:
With the rapid development of information technology and the Internet, the huge volume of user generated information has been collected by the providers of e-commerce and social networking services. This greatly facilitates the understanding of the user generated information. This talk will present our two main tasks in user generated information understanding. From the view point of user understanding, the aspect level sentiment analysis can find the users’ sentiment polarity towards a specific aspect, and thus it helps provide a thorough analysis of the users’ interests. From the view point of data understanding, sequential recommendation aims to capture the user’s long-term and short-term preferences from the user’s historical interaction data, and thus can greatly enhance the recommendation performance.
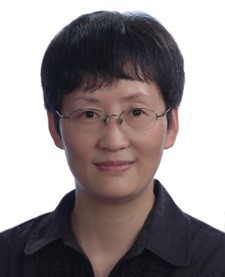
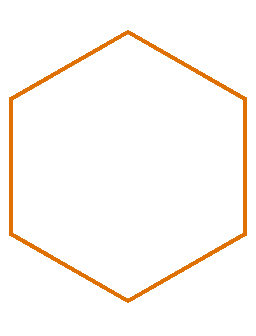
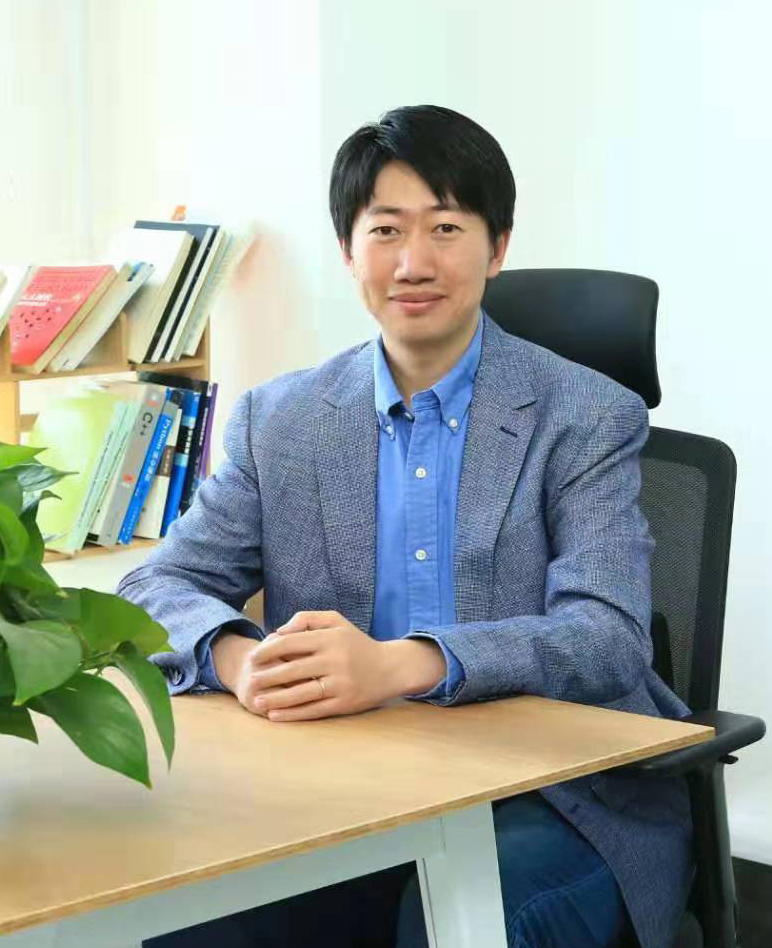
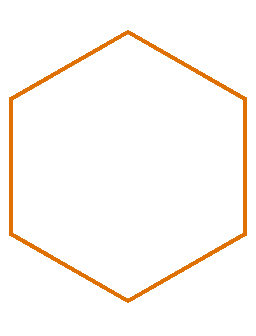
丁效 (哈尔滨工业大学 助理研究员)
丁效, 哈尔滨工业大学助理研究员/硕士生导师。主要研究方向为人工智能、自然语言处理、文本推理和事理图谱。2016年获得哈尔滨工业大学博士学位,已在AAAI、IJCAI、ACL、EMNLP、TASLP等人工智能领域的著名国际期刊和会议上发表相关论文20余篇。承担国家自然科学基金青年项目等省部级以上项目四项,参与国家重大科技基础设施建设项目、“新一代人工智能”重大项目、国家自然科学基金重点项目等多个科研项目。荣获黑龙江省科技进步二等奖、全国青年人工智能创新创业大会三等奖等荣誉。担任中国中文信息学会社会媒体处理专委会秘书、常务委员,语言与知识计算专委会委员,中国中文信息学会青年工作委员会委员。
报告题目:事理图谱的构建及应用
报告时间:14:15—15:00
报告摘要:
知识图谱在各个领域精耕细作,逐渐显露价值,但是现有的知识库普遍是以“概念及概念间的关系”为核心,较少记录“事理逻辑”相关知识,事理逻辑(事件之间的演化规律与模式)是一种非常有价值的人类知识,挖掘这种知识对我们认识人类行为和社会发展变化规律非常有意义。因此本次报告介绍一种新型的知识图谱形式:事理图谱,它是一个事理逻辑知识库,描述了事件之间的演化规律和模式。结构上事理图谱是一个有向图,节点代表事件,有向边代表事件之间的顺承、因果、条件和上下位等逻辑关系。本次报告重点介绍事理图谱的定义、构建、推理及应用。
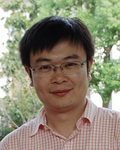
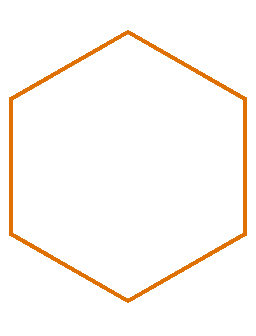